Scientific Data Management: Best Practices to Achieve R&D Operational Excellence
- john87833
- Jul 29, 2024
- 13 min read
Updated: Oct 17, 2024
2024
By: John F. Conway
Chief Visioneer Officer
20/15 Visioneers
Sponsored by: Pinnacle Technology Partners (PTP)
Introduction
Drug and Therapy Research and Development is a challenging and complex endeavor that demands efficient Scientific Data Management (SDM).
SDM comes with its own challenges with regard to importance and priority in startup organizations, especially when there is the overhead of investment to prove their science and get a molecule(s) or therapy into clinical trials as quickly as possible. Most investment models and stages depend on proof of concept and milestones, requiring prompt gathering, structuring, analysis, and presentation of data. In larger established biopharma, the challenge is digging out legacy data and approaches and reconfiguring outdated infrastructure and environments. These companies are drowning in data, which has been likened to painting an airplane while it's flying. Fortunately, data headaches are avoidable in today’s cloud-ready world.
In the past, the three main causes for avoiding or neglecting early SDM were cost, level of effort, and, unfortunately, ignorance. It is now abundantly clear that today’s next-generation biotech and biopharma won’t succeed without proper SDM. The reasons are due to the inherent science complexity, the resulting data volumes, data diversity, the scale of experimentation, and the need for high contextualization. The latest multi-omics efforts and associated bioinformatics are clear examples of this. Without FAIR data (Findable, Accessible, Interoperable, Reusable) (Wilkinson), organizations can quickly become mired in data wrangling, larger than necessary data science groups and initiatives, experiments, and testing that is not reproducible, and lastly, failed AI/ML initiatives. According to recent experiences and a report by Price Waterhouse Cooper and the European Union, unFAIR data and these associated problems cost the life sciences R&D industry in Europe tens of billions of Euros a year (European Commission and PwC). Our experience has shown the cost to be significantly higher in North America. Fortunately, unFAIR data and the associated problems are solvable through proper SDM. When SDM is done correctly, the efficiency gains in their R&D organization result in:
Scaling the organization (not just getting bigger but constantly improving with the resources at hand, e.g., not hiring data scientists as band-aids because the internal data environments are broken)
Data Efficiency, e.g., A major reduction in data wrangling
Reduced IT expenditure
More science, discoveries, and insights
Model- first or in silico-first, AI/ML readiness
Thus, when implemented properly, SDM effort paves the path to R&D operational excellence. Fig. 1 This operational excellence is necessary for industrialized science teams to closely work together to solve very complex problems. In this article, we will begin by describing the scientific data management lifecycle and move to an in-depth discussion on best practices and approaches that organizations can adopt to avoid mistakes in SDM efforts that can be detrimental to their scientific progress.
“When executed with precision, scientific data management (SDM) efforts not only chart the course to R&D operational excellence but also become vital for industrialized science teams to seamlessly collaborate in solving exceptionally complex problems.”

Figure 1- SDM-The Path to Operational Excellence
The Critical Importance of a Scientific Data Culture and Strategy
We can’t emphasize the need for the right data mindset enough. All scientists in 2024 and beyond should consider data an asset! Data is another form of currency and should be guarded and managed as such. R&D organizations invest millions to billions in research; that money, coupled with scientific intuition and enormous amounts of experimentation, produces immense amounts of scientific data. Fig. 2
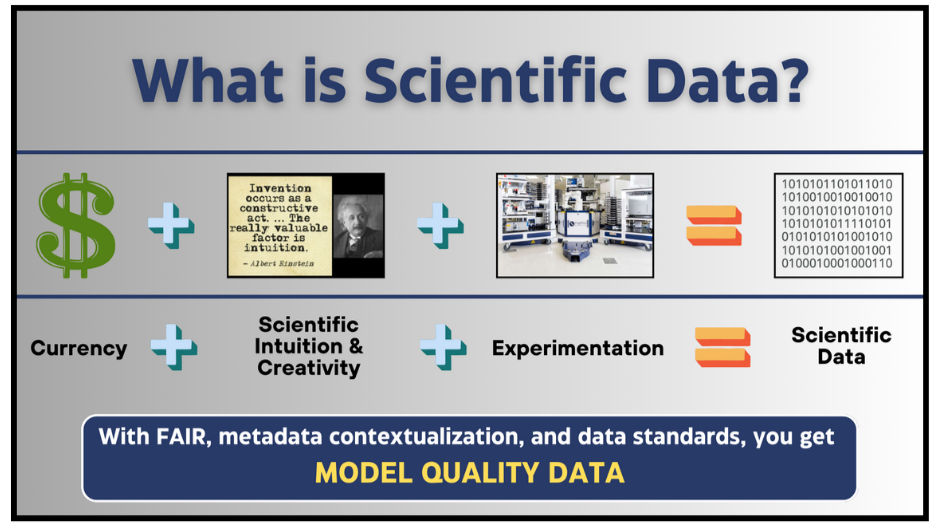
Figure 2- Scientific Data is Another Form of Currency
20/15 Visioneers has estimated that the biopharma and healthcare space will produce greater than 3 Zettabytes (a trillion gigabytes) of data in the next two years.
The organization owns the data, pays scientists and researchers to produce it, and expects them to protect and value it. Unfortunately, too many scientists and researchers were not trained to think this way; however, there is a multi-part best practice cadence to achieving a highly effective SDM at every data-driven R&D organization that starts with treating your data as an asset. If an R&D organization glosses over this and goes right into a scientific data strategy, they will most likely fail. In the past ten years, 20/15 Visioneers have experienced and confirmed that ~85% of Digital transformations have failed and not met their objectives (Bonnet; Bonnet). Our experience shows that this failure can be heavily blamed on a lack of culture change and inadequate change management.
Ultimately, their organization’s future depends on their data being FAIR and highly shareable (with the correct permissions). Organizations won’t be able to take full advantage of this data in their AI/ML efforts without FAIR data and a proper data culture. Once they set their data culture, it’s time to build their scientific data, process, and decision strategy. An organization that can accomplish this will be well positioned for a model-first/in silico-first approach to their R&D efforts, equating to at least a 40% efficiency gain in our R&D experiences.
The Scientific Data Lifecycle: the Core of SDM
Like all data, scientific data has a lifecycle. Having the right data culture with the right strategy (business rules, governance, stewardship, etc.; Fig. 3) upfront builds a foundation that supports future scientific data use from primary to secondary use, e.g., repurposing data to investigate new target molecules as they are identified. A properly executed SDM strategy also eventually allows tertiary data use for running efficient AI/ML. Currently, too many companies are rushing into AI/ML approaches without the proper foundation in data culture to support the evolution of data use. Without this foundation, the system lacks model-quality data to drive a model-first or in silico-first approach to R&D, hindering scientific progress. The good news for startups is that you can implement the right scientific data culture and strategy before data gathering starts. This best practice will drive FAIR principles by adopting data standards, selecting scientific software and instrumentation that best meets your needs, driving compliance with business rules and governance, and preserving data culture through stewardship.
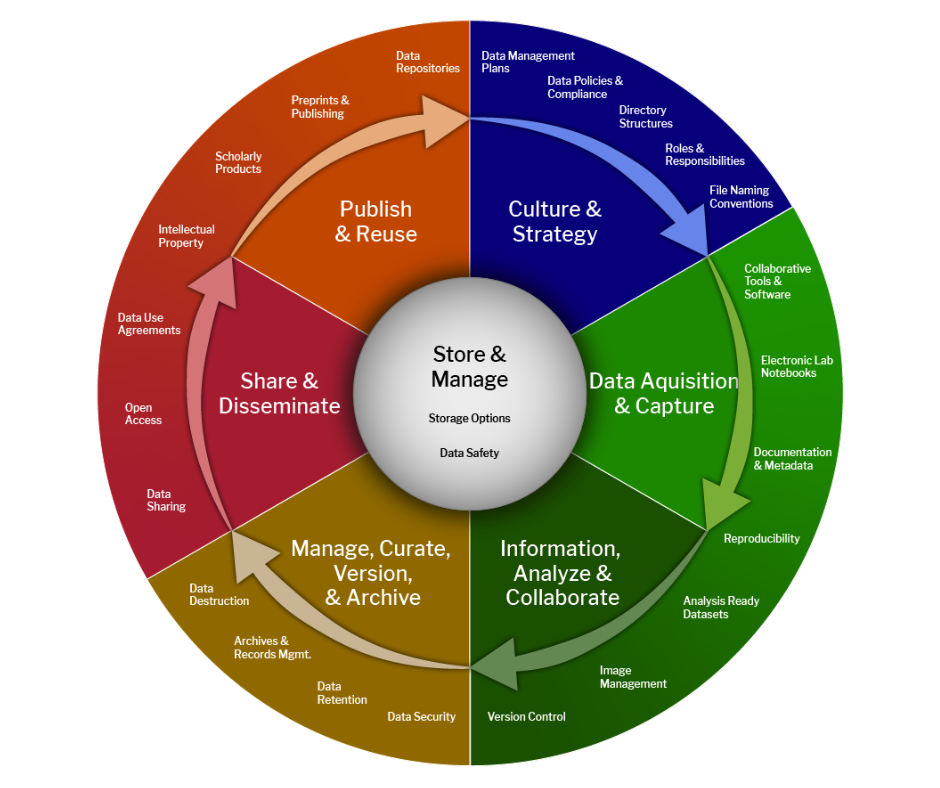
Figure 3- Comprehensive Representation of a Scientific Data Lifecycle and Associated Best Practices-edited (Cioffi, Goldman and Marchese)
The outer ring represents the needs and “best practices” in highly functioning R&D organizations. These typically cross the six high-level managed processes: Request, Sample, Experiment, Test, Analyze, and Reporting. When these processes are satisfied, R&D organizations tend to run more efficiently.
Since a comprehensive understanding of the scientific data lifecycle is key to the success of biopharma R&D, we will elaborate on the basic concepts outlined in Fig. 3. As this is a cycle, there are steps in the process of implementing an environment for ultimately publishing and getting reuses out of your data.
The first step in the data lifecycle, data culture, comes before any data is generated. A scientific data strategy - how data is optimally produced and how it will be efficiently consumed – is also critical in this stage. An organization must identify the right data culture and strategy to lay the foundation for any R&D efforts. Additionally, establishing data standards, directories, taxonomies, and ontologies are critical for data consistency. Lastly, compliance rules need to be outlined.
The second step is to collect data and associated metadata. This stage is the data acquisition and capture phase. Compliance with FAIR principles ensures that all future information and knowledge will have a basis of contextualization and location. A properly configured, templated, and integrated Electronic Lab Notebook (ELN) is also best to implement at this phase. An ELN allows scientists to streamline the capture of their scientific method, collaborate efficiently with scientific rigor, and drive reproducible science.
The third step is the information, analysis, and collaboration phase. Analyzing data generates information through appropriate data analysis choices, including exploratory analysis. Central to analyzing data is collaborating to gain perspectives that support decision-making, and group efforts are often needed to support the massive amount of generated data.
The fourth step is to manage, curate, version, and archive your data. Data structuring and information sharing are being used for data-driven discoveries and insights. Data should be versioned appropriately. Special attention should be paid to data security, retention, archiving, and destruction.
The fifth step is to share and disseminate your data. Central to this step is ensuring your data are FAIR in the primary, secondary, and tertiary phases.
Lastly, the sixth step is to publish and reuse the data. This requires using advanced environments and mechanics like data repositories that support AI/ML, intellectual property considerations, and scholarly products such as open-access publications.
When proper SDM is incorporated for each of these six steps in the data lifecycle, you will optimize the storage and management of your data, including ensuring data safety and allowing for operational efficiency in your biopharma R&D endeavors.
Cloud-First Principles
With proper guidance, experience, and skills, cloud computing and SaaS can minimize some of the efforts and expenses associated with SDM and data lifecycle investment. With a cloud-first model, there is no need for internal data centers, servers, or on-premises installations and the accompanying IT support.
In today's highly externalized and collaborative R&D world, a Cloud-First approach is a Best Practice.
As the company scales, you can seamlessly scale the cloud-based IT infrastructure. There are no cloud migrations, and your return on investment (ROI) is more extensive because you don’t have to invest in the on-premises environments and the FTEs to manage it. However, as with on-prem IT infrastructure, it is important to manage and stay on top of cloud footprint and environment(s). Without proper oversight, it is possible to waste money and end up with an unFAIR environment, but in the cloud. Recent projects in optimizing how and where cloud infrastructure & compute environments are used have led to significant cost savings and improvements in FAIR data quality.
These are just a few examples of why you need proper scientific data management practices. Founders and organizations might only get one shot at their startup. When organizations become deficient in scientific data management, operational efficiency will suffer, and they will even fail to convince their investors why they should invest in the next round.
Data Capture/Acquisition
A scientific data strategy dictates how to capture scientific data with the proper contextualization or metadata capture level. There is a level of complexity here, as mentioned before, not only with the data volume, diversity, and scale but also with the diversity of the instrumentation/software and the ability to integrate with these instruments and software in an intelligent and hopefully seamless way. An ELN is a perfect example of a data capture strategy where business rules are needed. These rules should address which data types and volumes need to be managed in a separate environment and referenced or linked back to their ELN experiment, which types of data are to be stored directly in an ELN, and how immutable raw data will be protected. An organization’s data strategy dictates the storage location for this raw experimental and contextualized data.
Vendors and tools like AWS Storage Gateway or GPU NAS are often utilized in the cloud. If an organization’s scientific business process workflow requires on-prem and time-bound storage, then setting up the proper network to manage the organization’s needs, like data to/from the cloud, cloud networking, and bench to processing, is imperative. Without proper management of on-prem to cloud inefficiency, it can cost significant money and time. Solutions, like Edge Computing, in some of these situations, are warranted as they are performant and closer to the end user. It is an outstanding case for business process mapping and understanding the details!
Data Storage Layer
Many organizations need help understanding best practices for data storage. This includes an understanding of the data diversity, how they get stored, how they are accessed and interacted with, and what structure is required.
Computational Needs are Dictated by the Science and the Data
Whether a global biopharma or a startup, R&D organizations must provide the needed computational tools and high-performance computing (HPC) to the right platform and network to support the data producers and consumers. Poor network or computational performance is a sure way for scientists and end users to lose confidence in IT and informatics groups. R&D organizations need computational-intensive groups to work with IT to acquire or build advanced or specialized HPC environments. These HPC environments come in 3 flavors: on-prem, cloud, and hybrid. These environments will continue to evolve as technologies and capabilities like NVIDIA GPUs and, someday, quantum computing as it continues to improve and become mainstream.
Building bespoke computational tools must be carefully considered, as they can lead to crippling technical debt. A common problem encountered is the need to salvage a research group after its leader leaves the company. After this departure, the IT department often struggled to make heads or tails of the group’s data processes, as it failed to comply with an organizational-level data strategy.
Data Silos
Data is typically captured in a siloed way and then migrated to a FAIR data environment using a plethora of data ingestion tools, pipelines, and workflows. As the data acquisition section mentions, a business process understanding and optimization exists here. While there may be reasons to keep data siloed, e.g., extreme data security, it doesn’t negate the need for contextualization, versioning, and adherence to other business rules dictated in the scientific data strategy. Technologies like data federation and virtualization can map and present data across databases and simulate a data lake-like environment. When warranted, data lakes can be a central storage location for large amounts of heavily contextualized data. It is a central place to access and find data.
Unlike federation or virtualization of data, physically moving large amounts of data back and forth from the cloud can get very expensive. Deep business analysis will provide organizations with which data needs quick access, local or continual analysis due to file size, and whether data should be migrated or archived. Continual optimization of cloud computing and storage can save organizations serious money. AWS intelligent tiering is an example. MemVerge, Domino Data Lab, and Wasabi are more examples. These tools can optimize computational spend by either moving compute jobs to lower cost ques or manage and control I/O costs, which we know can get expensive.
Data Structuring
Structuring complex data is critical in bioinformatics. Biological data is produced 24/7, 365 days a year, in most biopharma companies and CROs; however, processing and analyzing it comes with time constraints and limits. Bioinformatics pipelines are a critical part of this process in drug and therapy discovery today. The recent onset of multi-omics and the need to bring the omics data back together for insights and understanding has made clear data structuring a necessity.
Today, beyond the basic helper algorithms, lists, intervals, hashes, balanced trees, prefix trees, and many more, there is now a multitude of graph capabilities that enable the computational manipulation of the data and the ability to view and absorb it.
There are a handful of go-to solutions in this space. Scientists use aspects of software like Cell Ranger, Seurat, Picard, Star Aligner, etc. Different personas use tools like Nextflow and Airflow to create, deploy, and share data-intensive, highly scalable pipelines/workflows on most infrastructures. Using data structure is a remedy to remove bottlenecks with software. Being creative with code and efficient scientific data management avoids or prevents manual approaches or components, which always create bottlenecks. Kicking off jobs using AWS batch and Lambda scripts are more examples of game changers, allowing scientists to automate work that was previously highly repetitive and effort-intensive.
Data and Information Destinations
In Fig. 2, slices four and five essentially talk about where an organization’s data is going to be stored and curated. A critical step for R&D organizations, as they mature, is having FAIR scientific data in centralized Data Lakes, DataMart’s, or other structured environments so that scientists can use the data to perform initial analysis, exploratory analysis, and ultimately make decisions. AWS uses its S3 database technology as the core of its data lake technology. The levels of structuring can either be part of the data storage container, or the structuring can occur outside for specialized use like inter-project, intra-project, or in a specific program.
Scientific data curation and harmonization is an expensive endeavor but also necessary. Historically, metadata has been missed and imputation methods, with versioning, have been used to update missing metadata. The UK Biobank has many examples of the scale of total data management needed to harmonize data. The conversion of ICD codes to OMOP in the common data model took 6 months and involves a PB of data during the process. The final dataset is tiny relative to the intermediates. A larger scale example would be updating the original variant data from SNP chips reported in 2016 using the whole exome sequencing data collected by 2021 in the UK Biobank project. A series of new imputation models were calculated and tested based on the freshly collected whole exome sequencing data. The re-calculation of the models and the re-imputation of data involves more than 5 PB of data when including intermediate data forms. (Rubinacci).
One of the trickiest steps in curating the data is to harmonize naming conventions and identity refinements. For instance, the advent of long-range RNA sequencing reveals the exact splice forms of mRNAs that had been hidden previously. Thus, a historical gap in the data requires either imputation to bring the old, low-resolution forward for modeling or leaving the old data for model confirmation purposes. The emergence of new representations of genes to accommodate LINES, RNA splicing, and other RNAs will add to the curation challenge. There is tremendous value in accurately curated and harmonized data because this data can be highly complex, like multi-omics. If proper SDM has occurred, the highly structured, contextualized information can be used or easily earmarked for AI/ML, Generative AI, or LLMs.
Once data has been properly structured and curated, the data engineers and informaticians are ready to consume this data for secondary and tertiary use.
The Power of FAIR Data and Information
The truth is that Scientific Informatics is in its infancy. Many organizations are taking baby steps instead of operational leaps because of poor data culture. This has also contributed to making FAIR principles a reality and has proven difficult for many mature R&D organizations to achieve. One thing that the FAIR principles will provide is confidence in data. Today, because of the poor data culture that’s been established, too many organizations will find it easier to repeat experiments and testing because a) they can't source the original data, or b) they can find and access it, but they lack confidence in the data. The necessary metadata and/or versioning was missing to give the scientist just cause to accept the data's validity.
Now imagine the scenario in which SDM is done right, and the data are FAIR. The immutable data has a high confidence score as it has been used several times outside of primary use, scientists aren’t unnecessarily repeating experiments but instead using the FAIR data in their exploratory analysis, optimized pipelines, and algorithms, and lastly as training sets in their machine learning and next-generation AI approaches. That power is in efficiency gains and operational excellence, eliminating data wrangling and unnecessary repeated experiments. Furthermore, science is reproducible and traceable. All of this results in R&D Operational Excellence.
Conclusion
Each step in the scientific data management lifecycle will ultimately impact the organization’s ability to conduct its research efficiently. Cutting corners in one place will become their headache and technical debt in another. 20/15 Visioneers has successfully reduced this dysfunctional and technical debt, it can sometimes be as high as 75% of their R&D IT budget! The first phase in your scientific data journey is adopting a data-as-an-asset culture (become a data company), and the second is creating an effective and business rules-based scientific data strategy. Your next step is to define your processes with business process mapping and then take these living documents and create your scientific data management roadmap, which in today’s Life Sciences organizations is usually a cloud-first approach based on the capabilities that have been outlined in this white paper.
This approach guarantees your organizations will achieve FAIR data and processes, create reproducible science, minimize technical debt, and be positioned to take full advantage of an AI/ML strategy like Model-First or in silico-First. Attempting to prioritize AI/ML implementation without first addressing and enhancing data environments and organizational/data culture will most definitely result in failure, akin to challenges encountered in the digital transformation endeavors…
Comments